As technology becomes an increasingly important part of life science research, scientists rely heavily on methods such as digital PCR (dPCR) to collect data.
The problem? Instrument quality, user error and other factors can impact the ultimate data quality, leading to potentially unreliable or unintelligible output.
This article will outline what defines “good” quality data, how inadequate equipment or issues in the lab can compromise data quality and what researchers can do to ensure optimal dPCR data in every experiment.
Every scientific question relies on trustworthy and consistent data for answers. However, not every experiment yields data that leads scientists to valid conclusions. Because data quality is so foundational to science, it is vital that researchers understand what defines a “good” endpoint in every method they employ.
Careful experimental design, user expertise and well-maintained, high-quality instruments and reagents are all fundamental to generating “good” usable data that contribute to valuable insights. Without clear and accurate information, researchers can’t make conclusions that guide their subsequent experiments.
Digital PCR (dPCR) technology is an increasingly valuable tool in research throughout the life sciences. However, although dPCR has introduced new levels of sensitivity and precision, not all instruments and methods are created equal.
Without reliable instrumentation, quality assays and reagents, and proper use, researchers cannot count on this method to produce consistent and trustworthy results.
In this article, I’ll discuss what defines “good” versus “bad” data in such experiments, how instrument quality and other factors can impact data outcomes and why it’s so important to maximise data quality.
Ultrasensitive nucleic acid quantification with digital PCR
Thanks to an initial step involving partitioning the sample of interest into thousands of individual reactions, dPCR technology is highly sensitive. This can be done on a microarray, a microfluidic chip, in microfluidic plates similar to qPCR or, in the case of Droplet Digital PCR (ddPCR), within oil-water emulsion droplets.
After dividing the sample into these discrete reactions, each of which ideally contains one or several DNA strands, researchers can conduct a typical thermocycling reaction and observe fluorescence in samples containing the target nucleic acid sequence.
By quantifying the proportion of “positive” fluorescent samples versus negative ones and analysing with Poisson statistics, researchers can determine the original number of target DNA or RNA molecules present in the sample.
This addition to the family of PCR approaches offers several notable advantages compared with its traditional counterparts. Although qPCR requires researchers to generate a standard curve to interpret assay results, dPCR technology allows for the precise, absolute quantification of nucleic acids in a sample.
Additionally, eliminating the need for a standard curve reduces the risk of human error and makes these assays more sensitive to rare targets in complex backgrounds.1
dPCR can detect small fold changes, such as a change in copy number, with few experimental replicates. Digital PCR is also more robust than other methods, offering high tolerance to common PCR inhibitors.
What defines dPCR data quality?
If not all dPCR data are “good,” then what constitutes “bad” data? As discussed previously, dPCR analysis depends on a clear binary between the bands of “positive” sample partitions containing the target nucleic acid sequence and “negative” partitions without it.
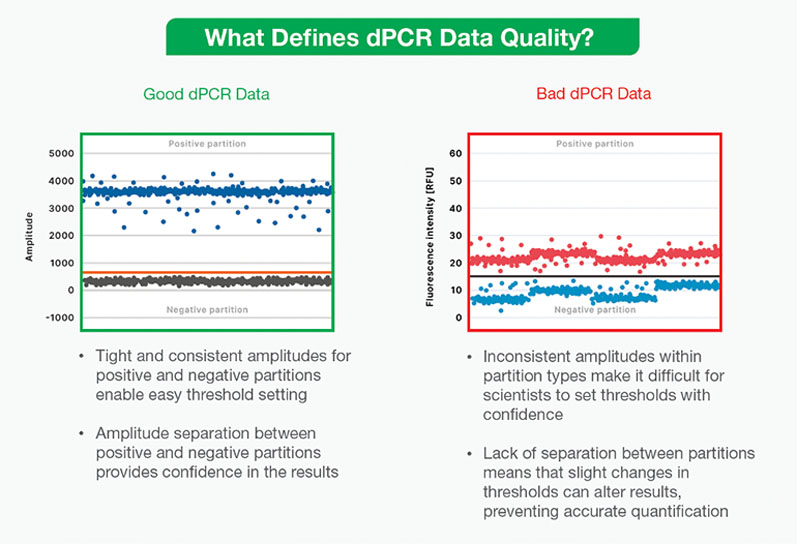
Setting a clear threshold to define positive and negative partitions is thus foundational to analysing dPCR data … but several factors can influence a user’s ability to make this distinction.
Noise and inconsistent amplitudes within positive and negative partition populations can make it difficult to confidently set a threshold for analysis. This can result from a number of issues: an inhibitor may have affected the reaction, the assay or instrument may be faulty or there could be cross-contamination in the sample.
If positive and negative partitions aren’t clearly separated, slight changes in the threshold can dramatically alter results and prevent accurate quantification. In addition, uncertainty in analysis can be an unnecessary stressor for researchers, forcing them to repeat experiments and waste precious time and resources.
Fortunately, understanding potential contributors to “bad” dPCR data can help to safeguard against these issues and maximise experimental success.
For good results, invest in quality tools and practices
Investing in good user training and high-quality instruments and assays can ensure that dPCR experiments yield accurate and reliable results. But what traits in a platform can compromise dPCR data? Here are some potential pitfalls to look for.
- Hardware instability: Instability in the dPCR instrument itself can lead to variation in partition amplitudes, compromising a researcher’s ability to confidently set thresholds. This can lead to false positive or false negative results and reduce the overall sensitivity of any assay being done.
- Random positive partitions: Random positive data partitions or “rain” appearing in a dPCR output can complicate data interpretation. These can appear when nucleic acid amplification occurs at varying rates across partitions in the dPCR assay, producing positive droplets that vary widely in fluorescence intensity. This makes it challenging to determine which partitions in the middle range of the plot are truly positive, reducing the assay’s sensitivity and limit of detection. Random positive partitions can result from low-specificity assays, noise from the instrument or poor sample partitioning.
- Noise: Often appearing as groups of negative data points above the threshold line, noise in dPCR can result from several issues. Bubbles or solid contaminants within the instrument can impede optical systems. However, even inferior optic technology can lead to reduced optical stability and, in turn, noisy data. Although a highly trained user may salvage noisy data, the manual process of making sense of results costs unnecessary time and effort.
- Cross-contamination: The cross-contamination of neighbouring samples can be uniquely challenging for dPCR analysis. Clarity isn’t the issue in this case; clear positive partitions appear above the threshold, but these points may result from another reaction. The target nucleic acid has migrated from one sample to another, producing a misplaced positive signal. Cross-contamination of samples can occur because of user error, environmental factors or instrument-induced sample dispersion. Although cross-contamination can occur in assays run on microarrays or chips, this risk is minimised in oil-water emulsion ddPCR assays because samples are completely separated from one another by oil.
Inconsistent and unclear data caused by any of these issues can quickly turn ddPCR technology from useful to burdensome for researchers and jeopardise the success of their experiments.
In addition, low-quality data can force users to make case-specific decisions when interpreting results, introducing an additional variable into their research.
Whether you’re conducting basic exploratory research or interpreting key clinical findings, purchasing quality dPCR instrumentation and assays is an investment in your future work.
The right tools take your research further
Every researcher’s demands are unique, but all are alike in the need for clear, consistent and accurate data to yield experimental insights. Choosing the right dPCR tools will prevent unnecessary stress and uncertainty in future experiments while maximising workflow efficiency.
In addition to selecting a dPCR instrument with high-quality optics, a stable optical bench and consistent sample partitioning, users should ensure that their system is compatible with a wide range of kits, reagents and assays.
By investing in a quality system, researchers can eliminate the additional costs of repeating experiments owing to unclear data. Across every application, a versatile and reliable dPCR instrument can yield good data, capturing the nuances of nucleic acid quantification and bringing users closer than ever before to absolute precision and accuracy.
Reference
- www.bio-rad.com/en-uk/life-science/learning-center/digital-pcr-and-real-time-pcr-qpcr-choices-for-different-applications.