By now, the scientific community not only shares a common understanding of the term big data, but is also enamoured with the sheer power of harnessing massive quantities of information. From the perspective of R&D, this new era of research can power real progress in solving difficult disease states. The most potent use of these mind-boggling datasets is likely to be in oncology. Thanks to the broad reach of the International Cancer Genome Consortium (ICGC), The Cancer Genome Atlas (TCGA) has amassed profiles of approximately 10,000 tumours. In total, the project has catalogued 10 million cancer-related mutations. All areas of cancer research have benefited from this immense undertaking.
But questions arise: once we have all this data, what do we do with it? And, as the era of big data now intersects with the era of personalised medicine, how does the life science community maximise this overlap?
As the era of big data now intersects with the era of personalised medicine, how does the life science community maximise this overlap?
Aside from the TCGA multi-year cataloguing initiative, other projects are in the pipeline to harness data-gathering and sharing. Other good quality data is available, albeit from geographically and technically disparate sources, which makes the tools and technologies that harvest meaningful information critical. How efficiently can we access, aggregate, mine and extract the right fodder for an individual workflow? Not to mention user-friendliness and the reduction of false positives and negatives. The next step is to put it all together.
The number of stakeholders who are dependent on huge, yet narrow, datasets continues to grow. In the life sciences arena, companies in pharma, biotech and diagnostics, as well as government agencies, academia, medical centres and research organisations, are drawn towards increasing inter-dependence through huge packages of data. At the same time, aggregations of genetic, proteomic and other, yet no less vital, patient information are revolutionising medicine and diagnostics.
All the data in the world, however, will not help that one patient with a very specific genetic profile unless it is absolutely the right data.
Narrowing the focus
New models for discovery of therapeutics for a variety of diseases and conditions continue to emerge, thanks in part to the audacity of scope of mapping projects like TCGA. These models are forcing change in the way new drugs are discovered and developed. While they may originate from different sources, they have critical components in common.
- Understanding disease: Big data has kindled the growth of ‘-Omics’ study. This method is vastly better than trying and failing to understand the molecular level structure of disease.
- Target selection: Modelling software that predicts the correct protein to structure relationship is key to choosing the correct target the first time.
- Target validation: Drug bioactivities that predict human response are tested via simulations, a safe and effective way of improving on older validation models.
- Discovery of molecules: Living systems are genetically engineered using high-throughput screenings to target specific diseases, which bolsters compound identification and candidate molecule synthesis.
- Optimising leads: Structural mapping technologies that are novel and flexible allow for rapid alterations and molecular structure customisation.
- In vitro testing: Lower risk preclinical testing for safety and efficacy is improving our understanding of physiological drug responses in animal models.
Drilling down to and addressing the characteristics of individual patients can enhance the ability of doctors to correctly and quickly treat the exact disease manifestation in each patient – all while bolstering the ability of a broad community of doctors to treat other patients more effectively and cheaply – thanks to big data.
To be sure, collected data from early stage R&D is still valuable in later stage drug development (including clinical trials), once it is properly organised and accessible. These trials are a valuable research tool; an additional way that expensive, up-front investments can still pay off for many years after the initial expenditures. Real-world patterns become clear too, and help in anticipating and mitigating risk through better pharmacovigilance.
A perfect storm is propelling life science towards a new age of breakthroughs. Medicine and diagnostics are converging with evolving R&D models. Personalised medicine technologies are becoming affordable and available. What is required is more sophisticated gathering and application of this vast pool of information. Curation is key: if the data is catalogued and retained in elegant, thoughtful and organised ways that withstand the test of time, future researchers need only query the aggregated knowledge to meet their needs.
More than 1.7 million individuals in the US have a cancer diagnosis, yet only 3% of those patients participate in clinical trials
Consider this: more than 1.7 million individuals in the US have a cancer diagnosis, yet only 3% of those patients participate in clinical trials – a time-honoured method for collecting data on a national scale. One exciting development in this space is the American Society for Clinical Oncology’s evolving CancerLinq portal. Still under development, the site will aggregate patient data with personal information stripped away, to collect massive amounts of clinically relevant data to supply doctors with a clearer path to treatment option. A lone patient with a rare genetic mutation will not be alone in diagnosis and treatment; anonymised data from similar patients hundreds of miles away will point towards treatment options where once there were none.
Clinical trials of significance do produce key data. For example, the Lung Master Protocol trial (Lung-MAP) is a multi-drug, multi-arm, biomarker-driven squamous cell lung cancer clinical trial that uses Foundation Medicine’s genomic profiling sequencing platform to match patients to one of five investigational treatments. This large-scale screening/clinical registration protocol is a public-private collaboration among government, not-for-profit and for-profit organisations that may serve as an important model for future drug registration trials.1 In the UK, the National Lung Matrix trial is a similar initiative for patients with metastatic breast cancer.2
However, collecting this data is one thing, analysing it is quite another. There is a significant challenge that will be faced by any entity that tries to use such enormous constellations of data. For example, the TCGA is comprised of 20 petabytes (1015 bytes) of data – a large, unwieldy body of information that needs tremendous computing power to look at as a whole.
Good data drives good decisions
Scientists can now use biological decision support tools to analyse their experimental data in the context of published results from the literature and from clinical trials. This process helps them to find patterns and possible causalities of disease. Many times, it is the most novel of relationships that provides the critical insight to the impact of diseases. With robust analytical solutions, researchers can incorporate multiple datasets, including gene expression, proteomics, protein-protein interactions, cell processes, disease mechanisms, treatments and functional drug classes, to identify meaningful associations between targets and molecules.
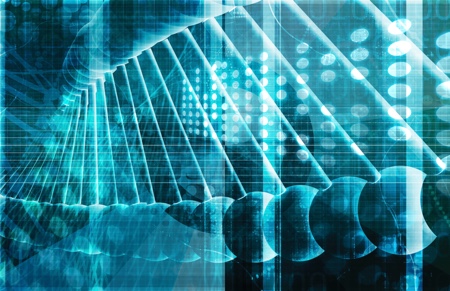
There is progress, but no magic bullet technologically. Some tools such as Pathway Studio, from Elsevier R&D Solutions, use a combination of information from a variety of research modalities to provide comprehensive and reliable information. Tools that enable the integration of information from DNA/RNA screens, pathway analysis algorithms and text mining, allow scientists to build more reliable molecular networks and gain new insights into disease mechanisms and potentially new therapeutics.3 Powerful analytics reinforced with structured taxonomies that aggregate, normalise and integrate data are setting the stage for improved decision-making that has a positive impact on patient care.
Every stakeholder in the life science space is driving the big data revolution in its own important way. With the influx of patient-centric data, such as that gleaned from electronic medical records and mobile monitoring, which is now being shared across the country and the world in realtime, patient care is undergoing an important transformation.
Every stakeholder in the life science space is driving the big data revolution in its own important way
Even though massive amounts of data may necessarily be available, honing in on the exact pieces that are relevant is the next big step. In the future, the TGCA may next look more closely at sequencing, which may uncover even more mutations, or turn towards exploring the ways that the mutations that we know about have an impact on cancer’s progression.4 Either way, data generation and analysis will continue to dominate.
With such enormous amounts of data, however, traditional data frameworks and tools cannot deliver the solutions that life scientists need. To increase R&D productivity and ultimately enhance patient care, our community needs better management systems and next-generation analytics to unleash the potential of big data.
References
1. SWOG Study Update, http://www.swog.org/Visitors/newsletters/2014/04/index.asp?a=s1400; New gene tests may give cancer patients a quicker path to treatment; http://www.foxnews.com/health/2014/06/06/new-gene-tests-may-give-cancer-patients-quicker-path-to-treatment/
2. Charlotte Harrison, 2014. Nature Reviews Drug Discovery 13, 407
3. Clinical Interpretation and Implications of Whole-Genome Sequencing JAMA. 2014;311(10):1035-1044. doi:10.1001/jama.2014.1717
4. http://www.nature.com/news/end-of-cancer-genome-project-prompts-rethink-1.16662