Pharmaceutical companies are looking into the next paradigm to deliver the promise of new treatments and build superior competitive advantage.
With the emergence of data analytics solutions, the transformation from a reactive to a predictive supply chain brings the potential for a better patient-and-caregiver experience. However, gaining confidence and know-how in the new predictive models is a prerequisite to the major capability building effort required.
To enable such models, companies need to develop advanced analytical models along with the ability to understand the causes and effects that will make their predictions successful. There are important opportunities in transforming operations and industrialising those solutions that address new supply chain needs, which will ultimately allow the deployment of new models of care.
Evolving imperatives and statistical biases
Distribution and drug consumption models are rapidly changing with the emergence of new business avenues based on individualised medicine, combination therapies, device/drug convergence, etc. These new models create an enhanced patient-and-caregiver experience but have major consequences for the supply chain in that they strongly increase its complexity.
Supply chain imperatives are evolving, moving from drug production and delivery systems that are limited in scope towards a versatile supply chain that addresses the needs of multiple stakeholders (caregivers, patients, insurance companies, etc.).
To face these new challenges and deliver the promised service levels, pharmaceutical supply chain managers need to develop new competencies. The traditional supply chain approach based on materials requirement planning has progressed to its limits.
From the patient perspective: the current supply chain creates frustration and complications in individualised treatments, or even fails to offer satisfactory performance with new treatment types (companion diagnostic, individualised dosage, combination therapies, etc.).
From an industrial perspective: as new treatments become available, new types of supply chains need to be established, with new actors as well as new technological and logistical systems. Managing these new treatments with traditional supply chain methods is costly and complex, with a high risk of non-compliance; for example, cellular and genetic therapies require the modification of cells collected from individual patients to create personalised medicines, and so extend the supply chain to include “new” players such as the individual patients themselves, or hospitals and other healthcare providers.
Yet, while creating new challenges in terms of regulatory compliance and scaling, the incorporation of these players also provides significant opportunities in terms of data collection for advanced analytics.
Furthermore, current forecasting approaches based on correlations encompass multiple statistical biases. Correlations are backward-looking and describe what has happened in the past. However, this has, as such, no predictive value as it does not in itself establish any causality.
One cannot be sure that because an event occurred in the past, it will happen again in the future (and with neither the same amplitude nor recurrence). Today, AI-generated predictions are based on probability, not causality. This works well in a number of situations, but much less so in others.
More specifically, correlations between variables do not mean that one of the results is caused by the other (causality). The failure of Google Flu Trends in 2013 illustrates this. Google aimed to predict the number of people with flu symptoms based on the number of Google search queries for flu-related topics. However, its results have been largely wrong; it estimated that 11% of the US population had had influenza in winter 2012–2013, which was approximately two times more than shown in official statistics (6%).
The bias of Google Flu Trends lies in the search for statistical patterns in the data, not for causality. Hence, when in December 2012 the news was full of stories about flu, many healthy people did Internet searches on flu, which influenced Google’s prediction.
With the multiplication of consumption and distribution patterns with small volumes and high variations, forecasting tools based on the extrapolation of past demand are malfunctioning. This causes problems for on-time product delivery, despite being critical for new treatments. The challenge when applying predictive analytics to the supply chain is to distinguish the random correlations from the ones that result from causal determinism.
The “why” behind the patterns
The challenge for companies is to develop new capabilities by identifying useful and necessary initiatives … without putting ongoing operations at risk by building service models on the basis of correlations that will not be sustainable in the future. The intelligent supply chain, based on predictive analytics and machine learning, is better at demand anticipation (SKU, quantity) and characterisation (localisation, service levels) by identifying and understanding the patterns influencing it, rather than projecting past demand. However, seeing patterns is not sufficient — understanding the “why” behind them is key.
Analytical tools allow pharmaceutical companies to ensure fluid and fast access to their treatments to clinicians. For new types of treatments, such as individualised therapies with “batch of one” drugs, operations should be transformed, and new capabilities are required (recruitment of data scientists, sociologists and AI specialists, among others). Furthermore, companies need to build the skills to adapt their factory and personnel planning to demand in real-time ... and to industrialise their approaches.
Joint development with experienced partners is required, of course, but not sufficient: injecting one’s own business and process expertise into the system is required to understand the causal relations influencing demand-and-supply patterns sustainably. The most advanced pharma companies have started to experiment with new forms of analytics to change the way operations are planned and service is delivered, but solutions are not yet at an “industrialised” level (Figure 1).
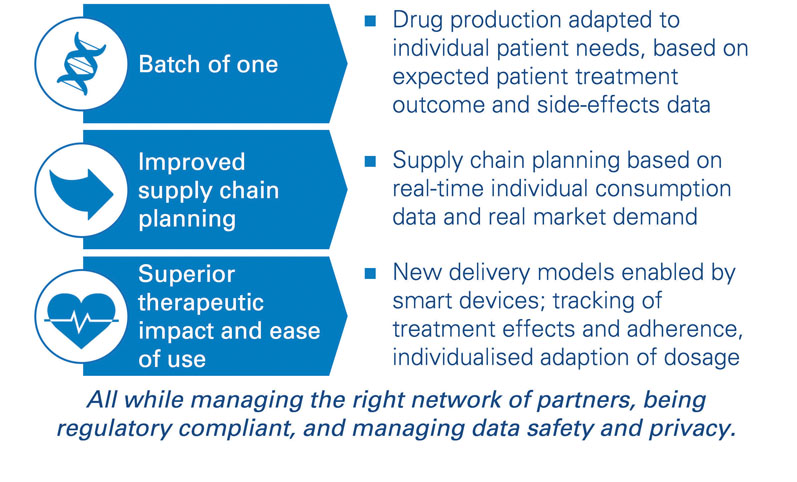
Figure 1: Forward-looking: can your supply chain keep up with digitally enabled therapies?
How advanced analytics can help
Advanced analytics allows for deep data analysis, which in turn facilitates the understanding of causal links. Leveraging those links, predictive analytics will be able to anticipate potential future states. The complexity of such models is to identify and select the links with predictive value.
The last levels of complexity are machine learning, in which a machine can accomplish certain tasks without prior programming, and prescriptive analytics, wherein systems identify the recommended choices through a complex web of options.
Data analytics pilots in other domains
Leading pharmaceutical companies are piloting data analytics solutions in different functional domains, essentially starting with R&D. They then investigate how those foundations can be exploited in other domains. For example, GlaxoSmithKline, in partnership with Exscientia, is using a machine learning-based platform to improve preclinical candidate discovery.
This technology designs new molecules, assesses them for their potency, selectivity and the ability to bind to specific targets, and uses a rapid “design-make-test” cycle to modify drug candidates according to the desired criteria.
Similarly, Pfizer and IBM have partnered to accelerate drug discovery in immuno-oncology thanks to Watson technology. Their collaboration aims to quickly analyse and test hypotheses extracted from large and broad data sources (laboratory and data reports, medical literature).
In a different context, we have seen the use of predictive analytics in production quality management: predicting from the external factors of the production chain which batches will be likely to deviate from the quality requirements and, consequently, significantly improve planning in the downstream part of production.
Predictive analytics and causal links
A prerequisite to mobilising investments and competences around predictive analytics is to develop confidence in the systems. Building on this idea, Arthur D. Little has developed a maturity model to evaluate the current situation and development priorities in the supply chain. Furthermore, our methodology allows thorough testing and leveraging of existing analytics pilots to outline key causal factors to integrate into the predictive supply chain:
- The first step is to better understand methodologies applied to various pilots, confirm their underlying hypotheses and identify whether other options are available.
- Secondly, pilot methodologies need to be tested on historical company data, and their results compared with actual history to exclude most of the “false positive” methods and identify repeated correlations. Those methods should be tested in an exhaustive, systematic and automated manner.
- At this step, the correlations have proved to be resilient with time; hence, causality can be assumed, but is not yet proven. A large number of “false positives” have already been excluded and a qualitative assessment of the repeated correlations is the best way to identify meaningful (with explainable links — no random coincidences) and useful causality links (when the variables can be measured).
- Ultimately, the stability of the methodology is pressure-tested, applying “derived laws” in Step 3 against all possible future scenarios to evaluate maximum variability and their implications through multivariate analysis.
Conclusion
In spite of being a nascent technology, predictive analytics is a top priority for healthcare supply chain executives.
Failing to prepare to leverage this technology is therefore not an option, and companies need to reflect on how to minimise the risks linked to initiatives in this field. Combining Arthur D. Little’s maturity model and analytical methodology provides a concrete starting point for leaders who want to develop new capabilities in the supply chain.